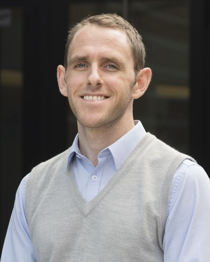
Submitted by Administrator on Wed, 30/05/2018 - 19:41
Tim Pearce will be attending the 35th International Conference on Machine Learning (ICML 2018). Tim is also attending a workshop, part of the conference, and presenting his work on 'Bayesian Inference with Anchored Ensembles of Neural Networks, and Application to Reinforcement Learning'.
Tim will be attending the 35th International Conference on Machine Learning (ICML 2018) and presenting his work on 'Bayesian Inference with Anchored Ensembles of Neural Networks, and Application to Reinforcement Learning'. The use of ensembles of neural networks (NNs) for the quantification of predictive uncertainty is widespread. However, the current justification is intuitive rather than analytical. This work proposes one minor modification to the normal ensembling methodology, which we prove allows the ensemble to perform Bayesian inference, hence converging to the corresponding Gaussian Process as both the total number of NNs, and the size of each, tend to infinity. This working paper provides early-stage results in a reinforcement learning setting, analysing the practicality of the technique for an ensemble of small, finite number. Using the uncertainty estimates they produce to govern the exploration-exploitation process results in steadier, more stable learning.