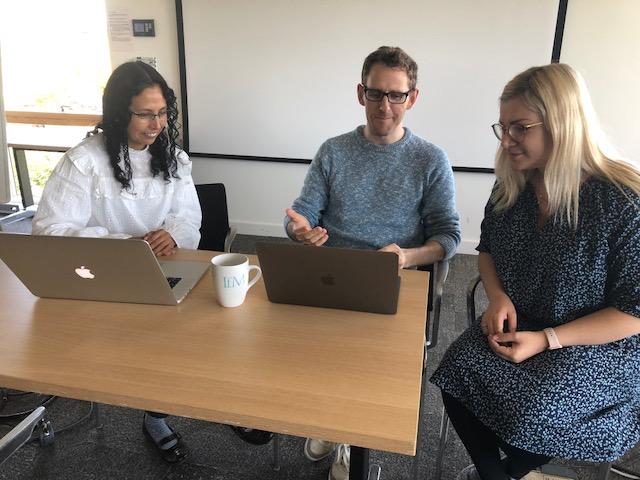
Submitted by Angela Walters on Mon, 11/06/2018 - 21:49
11 June 2018 - High-Quality Prediction Intervals for Deep Learning: A Distribution-Free, Ensembled Approach - by Tim Pearce, Mohamed Zaki, Alexandra Brintrup, Andy Neely
In this webinar, Tim discusses the February Alliance paper. Deep neural networks (NNs) have caused great excitement due to the step-changes in performance they have delivered in a variety of applications. However, their appeal in industry can be inhibited by an inability to quantify the uncertainty of their predictions. To take a prognostics example, a typical NN might predict that a machine will fail in 60 days. It is unclear from this point prediction whether the machine should be repaired immediately, or whether it can be run for another 59 days. However, if the NN could output a prediction interval (PI) of 45-65 days with 99% probability, timing of a repair could easily be scheduled. In this paper, we develop a method for doing exactly this - the quantification of uncertainty in deep learning using PIs. We derive a method based on the assumption that high-quality PIs should be as narrow as possible, whilst still capturing a given proportion of data. The method is general, applicable to any data-driven task where a continuous value needs to be predicted, and it is important to know the uncertainty of that prediction. Examples include the forecasting of precipitation, energy load, financial metrics, and traffic volume. The method is tested on ten real-world, open-source datasets. The proposed method is shown to outperform current state-of-the-art uncertainty quantification methods, reducing average PI width by around 10%.