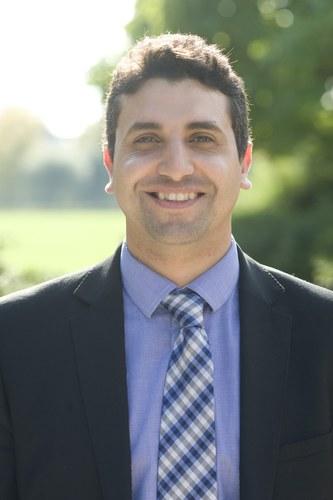
Submitted by Administrator on Thu, 31/05/2018 - 18:46
by Abdul Rauf Khan, Mohamed Zaki, Henrik Schiøler, and Murat Kulahci.
The paper was accepted at 'The 22nd Pacific-Asia Conference on Knowledge Discovery and Data Mining (PAKDD)', a leading international conference in the areas of knowledge discovery and data mining (KDD).
In this paper, Mohamed and co-authors propose a novel strategy for data mining based on partitioning of the feature space through Voronoi tessellation and Genetic Algorithm, where the latter is applied to solve a combinatorial optimization problem.
Classification is a major constituent of the data mining toolkit. Well-known methods for classification are either built on the principle of logic or on statistical reasoning. For imbalanced and noisy cases, classification may, however, fail to deliver on basic data mining goals, i.e., identifying statistical dependencies in data. In this article, we propose a novel strategy for data mining based on partitioning of the feature space through Voronoi tessellation and Genetic Algorithm, where the latter is applied to solve a combinatorial optimization problem. We apply the suggested methodology to a range of classification problems of varying imbalance and noise and compare the performance of the suggested method with well-known classification methods such as (SVM, KNN, and ANN). The results obtained indicate the proposed methodology to be well suited for data mining tasks in case of highly imbalanced classes and significant noise.