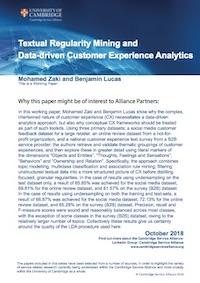
Submitted by Administrator on Tue, 23/10/2018 - 09:53
October 2018 paper on 'Textual Regularity Mining and Data-driven Customer Experience Analytics' by Mohamed Zaki and Benjamin Lucas
In this working paper, Mohamed Zaki and Benjamin Lucas show why the complex, intertwined nature of customer experience (CX) necessitates a data-driven analytics approach, but also why conceptual CX frameworks should be treated as part of such toolkits. Using three primary datasets; a social media customer feedback dataset for a large retailer, an online review dataset from a not-for-profit organization, and a national customer experience text survey from a B2B service provider, the authors retrieve and validate thematic groupings of customer experiences, and then explore these in greater detail using literal markers of the dimensions “Objects and Entities”, “Thoughts, Feelings and Sensations”, “Behaviors” and “Ownership and Relation”. Specifically, the approach combines topic modelling, multiclass classification and association rule mining; filtering unstructured textual data into a more structured picture of CX before distilling focused, granular regularities.
In the case of results using undersampling on the test dataset only, a result of 65.85% was achieved for the social media dataset, 69.61% for the online review dataset, and 61.57% on the survey (B2B) dataset. In the case of results using undersampling on both the training and test-sets, a result of 66.87% was achieved for the social media dataset, 72.13% for the online review dataset, and 65.29% on the survey (B2B) dataset. Precision, recall and F-measure scores were sound and reasonably balanced across most classes, with the exception of some classes in the survey (B2B) datatset, owing to the relatively larger number of topics. Collectively these results give us certainty around the quality of the LDA procedure used here.